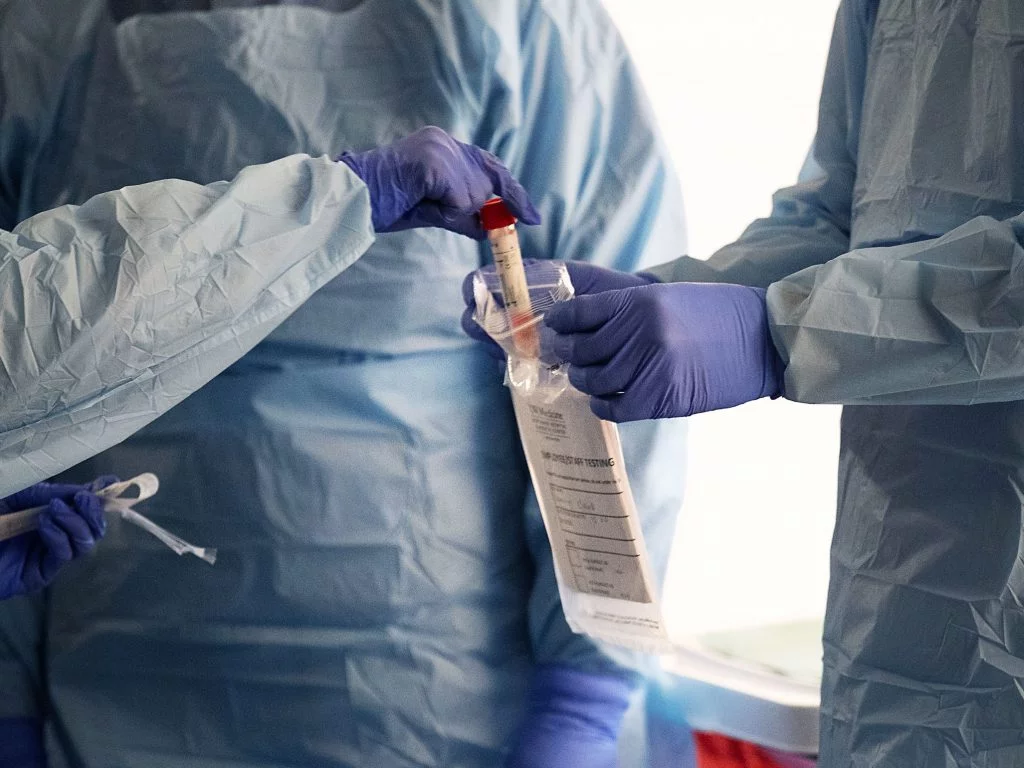
In a recent groundbreaking study, researchers have taken significant strides toward decoding brain activity, marking a substantial advance in the development of brain-machine interfaces. By harnessing an artificial intelligence (AI) image recognition algorithm, the team from Kobe University successfully predicted mouse movement based solely on brain functional imaging data, boasting an impressive accuracy rate of 95%.
The findings have been published in PLoS Computational Biology.
The motivation behind this study lies in the ongoing quest to decode neural signals, which is essential for the development of brain-machine interfaces. These interfaces aim to bridge the gap between the brain’s intricate signal network and external devices, potentially aiding in medical treatments and augmenting human capabilities.
The researchers utilized a cutting-edge form of brain imaging known as whole-cortex functional imaging, which captures the activity across the entire brain surface. Unlike traditional methods that focus on electrical activity in specific brain regions, this approach provides a more comprehensive view of brain dynamics.
The challenge, however, has been in processing these complex datasets, which contain an immense amount of information and inherent noise. Traditionally, significant preprocessing of the data was necessary to identify areas of interest and filter out irrelevant information, a labor-intensive process that could potentially overlook valuable insights.
The research team, led by medical student Takehiro Ajioka under the guidance of neuroscientist Toru Takumi, sought to overcome these hurdles. “Our experience with VR-based real time imaging and motion tracking systems for mice and deep learning techniques allowed us to explore ‘end-to-end’ deep learning methods, which means that they don’t require preprocessing or pre-specified features, and thus assess cortex-wide information for neural decoding,” Ajioka said.
Their innovative approach combined two distinct deep learning algorithms — one for analyzing spatial patterns and another for temporal patterns — applied to the whole-cortex film data of mice either resting or running on a treadmill. The AI model was then trained to accurately predict the mouse’s state based on the imaging data.
Remarkably, the model achieved a 95% accuracy in predicting the actual behavioral state of the mice without needing to remove noise or pre-define regions of interest. This was accomplished using just 0.17 seconds of data, indicating the model’s capability for near real-time prediction across different individuals, thus showcasing its potential for wide application.
What sets this study apart is not just its high accuracy rate but also its applicability across different individual mice. This universality indicates that the model can effectively filter out individual differences in brain structure or function, focusing solely on the relevant signals that indicate movement or rest. This feature underscores the potential for this technology to be adapted for broader, more diverse applications, including in humans.
Furthermore, the team developed a method to understand which parts of the imaging data were crucial for these predictions. By systematically removing portions of the data and observing the impact on the model’s performance, they could identify critical cortical regions for behavioral classification. This method not only boosts the model’s accuracy but also provides insights into the brain’s functioning.
“This ability of our model to identify critical cortical regions for behavioral classification is particularly exciting, as it opens the lid of the ‘black box’ aspect of deep learning techniques,” Ajioka remarked.
This study lays a robust foundation for the further development of brain-machine interfaces capable of near real-time behavior decoding using non-invasive brain imaging. By establishing a generalizable technique for identifying behavioral states from whole-cortex functional imaging data, the research opens new pathways for understanding how brain activity correlates with movement and behavior.
The ability to pinpoint which portions of the data contribute to the predictions enhances the interpretability of neural decoding models. This transparency is crucial for advancing brain-machine interface technology, potentially leading to more effective tools for medical diagnosis, rehabilitation, and even augmenting human capabilities through improved interaction with external devices.
“This research establishes the foundation for further developing brain-machine interfaces capable of near real-time behavior decoding using non-invasive brain imaging,” Ajioka explained.
The study, “End-to-end deep learning approach to mouse behavior classification from cortex-wide calcium imaging,” was authored by Takehiro Ajioka, Nobuhiro Nakai, Okito Yamashita, and Toru Takumi.